This report was obtained from the publicly-accessible government contractor wiki Semantic Community. For more information on its significance, see our article on the Catalyst system.
Intelligence Advanced Research Projects Agency (IARPA) Research and Development Experimental Collaboration (RDEC)
- 122 pages
- For Official Use Only
- June 21, 2008
(U) Catalyst, a component of DDNI/A’s Analytical Transformation Program, will process unstructured, semistructured, and structured data to produce a knowledge base of entities (people, organizations, places, events, …) with associated attributes and the relationships among them. It will perform functions such as entity extraction, relationship extraction, semantic integration, persistent storage of entities, disambiguation, and related functions (these are defined in the body of the report). The objective of this study is to assess the state-of-the-art and state-of-the-practice in these areas.
…
(U) The objective of this study is to assess the state-of-the-art and state-of-the-practice in entity extraction and disambiguation in the academic, the government, and the commercial worlds for potential use by the Catalyst Program, a component of DDNI/A’s Analytical Transformation (AT) Program. The AT Program is being executed by a variety of Executive Agents, managed by the DNI/CIO. We interpret this purpose to include all ancillary functions needed to develop an end-to-end system that takes as input unstructured data (primarily free text, with or without document-level metadata) and results in a knowledge base of entities (people, organizations, places, events, etc.) with attributes of these entities and the relationships among these entities. Thus, in addition to entity extraction and disambiguation, an eventual Catalyst capability will need functions such as relationship extraction, semantic integration, persistent storage of entities, and others to provide end-to-end functionality. Note that we are not defining what constitutes a Catalyst system, but rather what capabilities need to be performed by some processing component to result in the kinds of outputs envisioned for Catalyst, which are sets of semantically aligned, integrated, disambiguated entities of interest for a problem area.
…
(U) We assume that this generic processing starts with unstructured and semi-structured data, such as documents, images, videos, audios, signals, measurements, etc., as well as structured data, that are collected from a wide variety of sources by a variety of methods. We use the term resource to include all of these input data types. The objective of Catalyst’s advanced intelligence processing is to identify the entities—people, places, organizations, events, etc.—in the resources and what the resource says about the entities (the attributes of entities and the relationships among them). This information is made available to users (generally, intelligence analysts) so they can retrieve information about these entities and detect patterns of interest to their analysis mission.
(U) At a high level, there are three steps to the kind of intelligence processing related to Catalyst: (1) describing resources and making them capable of being processed, (2) semantically integrating entities of interest to a specific task (including disambiguation of these entities), and (3) processing the entities to produce some conclusion of interest. The figure below summarizes the three steps of intelligence processing. Each step is expanded upon below.
…
Share this:

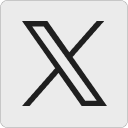


